Blog
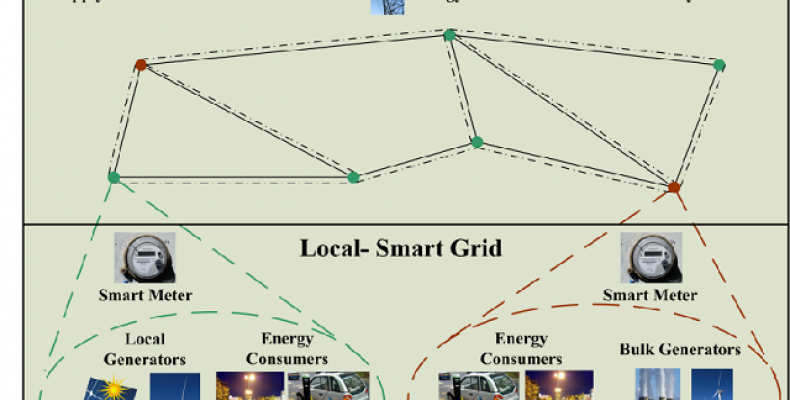
A New Way to Detect False Data Injection Attacks
False data injection attacks are becoming somewhat common these days, so finding preventive measures from such attacks is something that researchers are looking to do. This is exactly what researchers at Beijing Institute of Technology are aiming to do. Their technology was presented at the Annual Conference of the IEEE Industrial Electronics Society and outlines how to prevent false data injection attacks using a recurrent neural network.
False data injections hacks a problem?
Cyber attacks or false data injection attacks are a new way to cause chaos and havoc to people. They are particularly focused on disrupting power grids causing widespread disturbance to people living in those affected areas. False data injection attacks were seen in 2015 when a power grid was affected in Ukraine, leaving 230,000 people without electricity for several hours.
Researchers have found many different ways to prevent cyber attacks but none of them seem to be successful in preventing false data injection attacks on power grids. False data injection attacks are notoriously known for passing all modern surveillance and various other security techniques.
False data injections attacks works by allowing an attacker to compromise measurements taken by the grid which then disrupts its normal functioning even damaging the devices connected to it.
Detecting False Data Injection Attacks:
Researchers have been hard at work looking for ways to detect false data injection attacks. By doing so they could help prevent infrastructural damages to power grids caused by such attacks. Many of these ways so developed use machine learning tools.
These machine learning tools use both supervised and semi supervised learning algorithms to detect false data injection attacks.
Limitations to Using Machine Learning Tools:
While many of these methods made use machine learning tools they have various flaws and limitations that make them inherently unsuccessful in detecting a cyber attack.
Some of these machine learning tools so developed are prone to vulnerabilities associated with false data injection attacks themselves, while others cannot be trained to recognize an attack due to the limited amount of data that is available.
Overcoming the Problem:
Researchers have found a way to overcome these limitations in current methods by using a recurrent neural network or RNN for short. This RNN has several hidden layers with a fully connected layer at the top. This top layer has a linear activation function.
RNNs have been especially found out to be effective in time series forecasting and in anomaly detection. This makes it useful in detecting a cyber attack of the false data injection variety.
Studies showing the effectiveness of RNNs are what prompted researchers to look at using RNNs for detecting cyber attacks.
The RNNs used by researchers does not require labelled data to function which makes it easier to use in real world situations. Researchers applied this RNN to the IEEE-14 bus test system and got astounding results. The RNN was successful in identifying compromised measurements by using a small alarm rate or FAR for short.